Join¶
The Join task in Rulex Factory merges two datasets by their rows, creating a single table with all the data.
Merge operations can be used to produce a global dataset from on which in-depth analyses can be performed.
All merge operations are performed around the key attributes, which act as a unique identifier of each row in a table.
This task offers a full range of join options, as shown in the picture below: you can identify the join type, as the text graphically indicates how data will be merged.
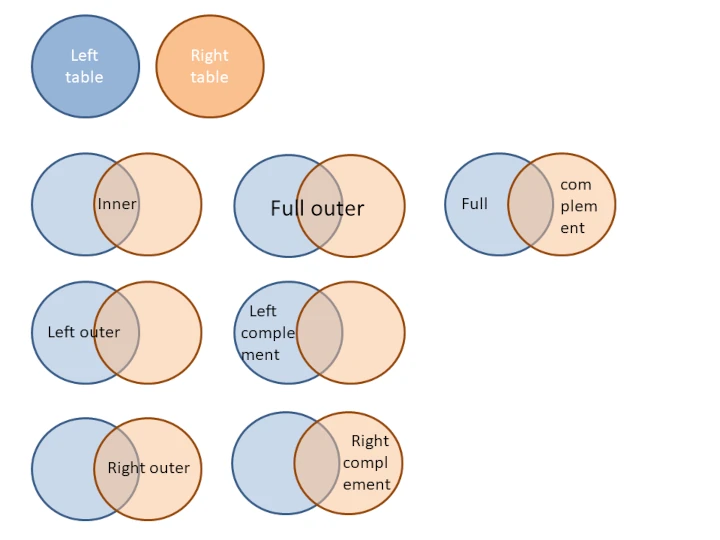
The Join task has a unique tab, the Options tab, which we can divide into two parts: the source attributes section and the Matching attributes for joining dataset section.
The source attributes section¶
In this section you will find three panels, each of them with a specific role:
The first two panels contain the attribute list of both the datasets involved in the join operation. They can be easily identified as their name is Dataset from <file_name>. These two panels are attribute list controllers, and they are linked to two underlying options. These options control the attributes which need to be added to the final dataset.
You can uncheck the attributes which aren’t needed in the outcoming dataset by clicking on the checkbox located next to them, or you can right-click on the attribute to open the attribute context menu. In this menu you will find the following options:
Invert, to invert the selection;
Check, to select the attribute;
Uncheck, to delete the selection on the attribute;
Check All, to select all the attributes;
Uncheck All, to delete the selection on all the attributes.
Tip
If you have the same attribute with the same name in both datasets, remember to uncheck one of the two, so that you don’t have two identical columns in the final dataset.
All the mechanisms to check/uncheck and modifying the underlying option are described in detail in the attribute controller overview.
The last panel contains all the join customization options available:
- Join type: select the combination method you want to use from the Join type drop-down menu. Possible types are:
Inner join: only the pairs of samples whose values of the key attributes values satisfy all the conditions listed in the matching attributes panels will be included in the final dataset.
Left outer join: the final dataset includes all the records from the dataset on the left even if the join condition does not find matching records in the right dataset.
If a row in the left-hand table does not match any row in the right-hand table the columns relative to the right-hand table will present empty cells.Right outer join: the final dataset includes all the records from the dataset on the right. If a row in the right-hand table does not match any row in the left-hand table the columns relative to the left-hand table will present empty cells.
Full outer join: the union of the tables produced by left outer join and right outer join is included in the final dataset.
Left complement: the final dataset includes all the records from the left-hand dataset that do not satisfy the conditions listed in the matching attribute panels. Since the rows in the left-hand table do not match any records in the right-hand table, the columns relative to the right-hand table will all be empty.
Right complement: the final dataset includes all the records from the right-hand dataset that do not satisfy the conditions listed in the matching attribute panels. Since the rows in the right-hand table do not match any records in the left-hand table, the columns relative to the left-hand table will all be empty.
Full complement: the final dataset includes all the records from both datasets that do not satisfy the conditions listed in the matching attribute panels.
- Missing policy: select the policy you want to adopt for managing missing values when evaluating the constraints on the matching attributes:
Missing values are considered normal values: missing values are considered missing, and are matched only with other missing values.
Missing values always satisfy equality checks: missing values are always considered as matching.
Missing values never satisfy equality checks: missing values are never considered as matching.
- Merge type: this option manages attributes with the same name (or the same position according to the Match columns by name options) that are present in both datasets. Possible merge types are:
None: if the attributes are not merged, a separate column will be created in the resulting dataset for the left-hand and right-hand dataset attributes.
Left fill: the value in the right-hand dataset overwrites the value in the left-hand dataset. If there is no value in the right-hand dataset for the attribute, the corresponding value in the left-hand dataset is kept.
Right fill: the value in the left-hand dataset overwrites the value in the right-hand dataset. If there is no value in the left-hand dataset for the attribute, the corresponding value in the right-hand dataset is kept.
Match column by: choose how you want to match columns, by Name (default value) or by Position.
The Matching attributes for joining dataset section¶
In this section, which is identified by the Matching attributes for joining dataset header, the join operations happen: you need to drag the key attribute for both datasets onto it. The key attributes are the attribute controlling the comparison which leads the join operation.
Once both attributes have been dragged onto this section, a connection line appears between each pairs. The connection yields two properties: the Operator and the Weight. These two properties establish the type of comparison will be performed between the two attributes connected by the line. By selecting one of the connection line, you can change these internal connection options on the right in the Edit match section. If no line are selected, the Edit match section is greyed out.
You can configure the connection by modifying the following properties:
- Operator: choose the match operator. The operators available are:
=: equal to.
!=: different from/is not equal to.
<: less than.
<=: less than or equal to.
>: more than.
>=: more than or equal to.
is substring of
is superstring of
is not substring of
is not superstring of
begins with
is the beginning of
does not begin with
is not the beginning of
ends with
is the end of
does not end with
is not the end of
Demerau-Levenshtein dist % is <=
Levenshtein dist % is <=
Hamming dist is <=
Longest common substring dist % is <=
Demerau-Levenshtein dist % is >
Levenshtein dist % is >
Hamming dist % is >
Longest common substring dist % is >
is the anagram of
is a word of
comprises as a word
is included (with gap) in
includes (with gap)
same primary phonetic of
same secondary phonetic of
some phonetic in common with
both phonetics in common with
Weight: define the weight of the attribute resulting from the merging operation performed.
Hint
The value chosen for each connection line are reported on top of the line
Note
Located at the bottom of the screen, these checkboxes allow users to set the missing values’ filling policy when using an outer or complement join type.
Insert right key value when missing: if selected, it fills the missing key attribute values with the corresponding key value in the dataset on the right. To perform this operation, you need also to uncheck the attribute located on the right in the source attributes.
Insert left key value when missing: if selected, it fills the missing key attribute values with the corresponding key value in the dataset on the left. To perform this operation, you need also to uncheck the attribute located on the left in the source attributes.
Example¶
- After having imported the northwind_customers and the northwind_orders datasets, we can state, by right-clicking on the import task and selecting Take a look, that:
- The northwind_customers dataset contains:
91 records
11 attributes (all nominal)
- The northwind_orders dataset contains:
830 records
14 attributes (of various types)
Add a Join task and link it to both the import tasks.
Compute the Join task. If the task is executed with the default options no attributes are present in the matching panels and therefore the row numbers are employed to combine datasets.
In this case the resulting table has 91 records with 25 attributes.
Note that the name CustomerID of the first attribute deriving from the right dataset has been changed to Customer_ID_1 to avoid a conflict with the name of the first attribute.
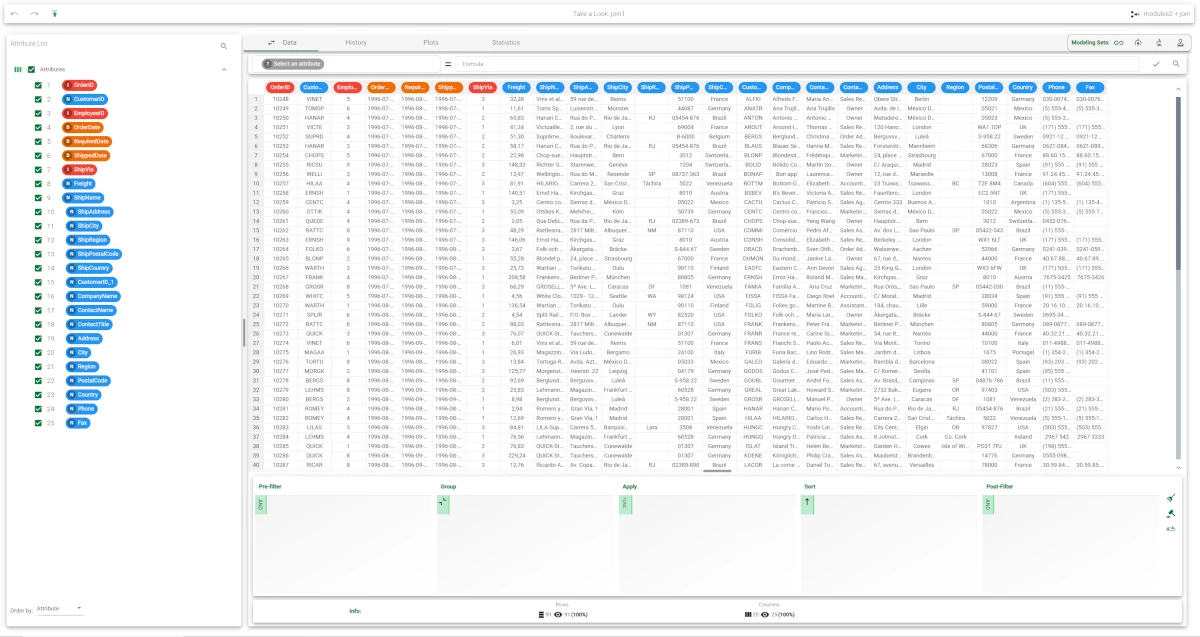
Open the Join task and match the CustomerID and City attributes from the dataset on the left with the CustomerID and ShipCity attributes from the dataset on the right, and set Inner join as the Join type.
The resulting dataset includes 817 records with 23 attributes.
Only 817 different values in the CustomerID/ShipCity attribute pair from the right dataset match the values in the CustomerID and City attribute pair from the left dataset.
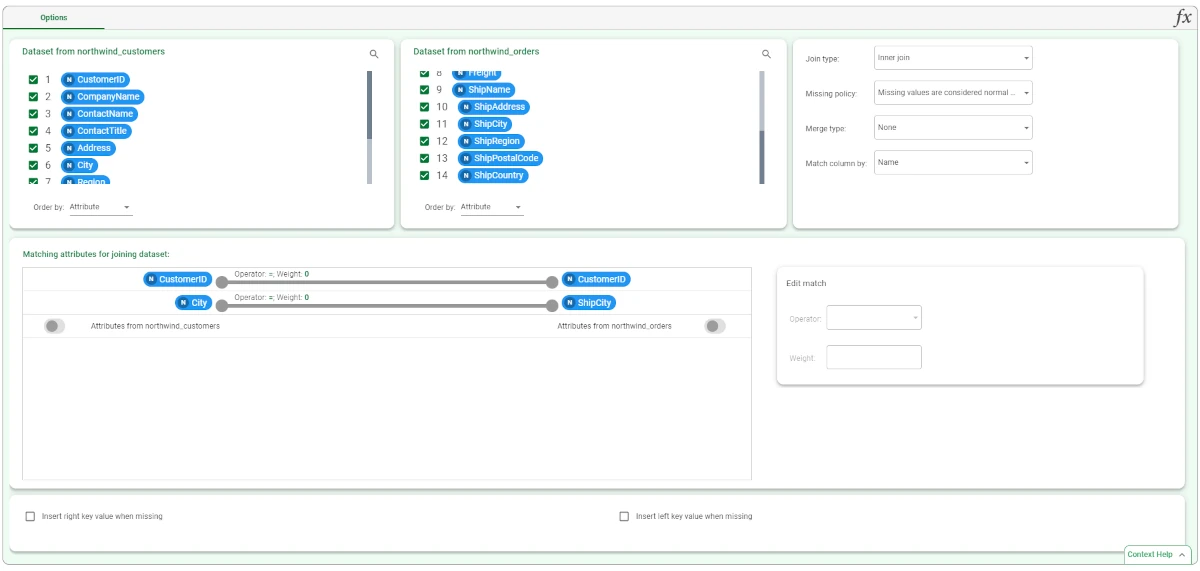
The resulting dataset includes 817 records with 23 attributes.
Only 817 different values in the CustomerID/ShipCity attribute pair from the right dataset match the values in the CustomerID and City attribute pair from the left dataset.
It can be visualized by linking a Data Manager task to the Join task, or by right-clicking on the Join task and selecting Take a look.
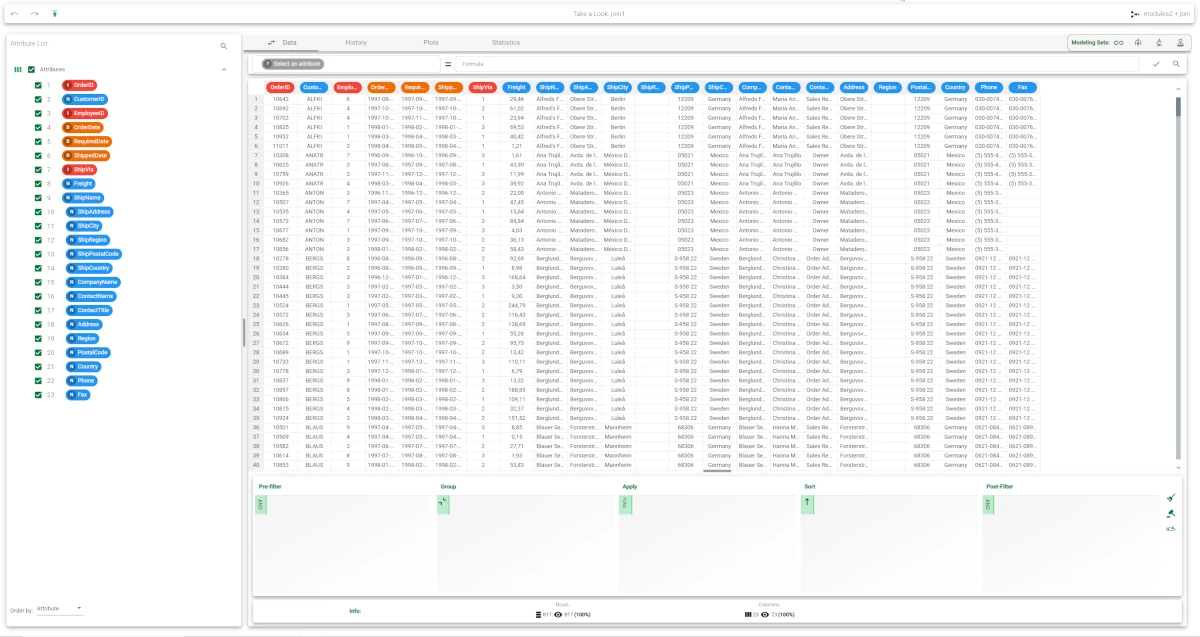